Finding Order in Noisy Markets: The Hedge Fund Journal
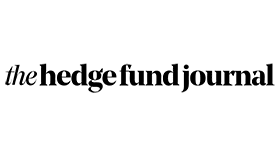
The following was prepared by a third party unaffiliated with Millburn. Please see the important disclosures appearing here (https://www.millburn.com/disclosures) and at the bottom of this page. PAST PERFORMANCE IS NOT NECESSARILY INDICATIVE OF FUTURE RESULTS. THE POTENTIAL FOR PROFIT IS ACCOMPANIED BY THE RISK OF LOSS.
The views and opinions expressed herein are those of the author and do not necessarily reflect the views of Millburn Ridgefield Corporation, its affiliates or its employees.
This document has been provided to you solely for information purposes and does not constitute an offer or solicitation of an offer or any advice or recommendation to purchase any securities or other financial instruments and may not be construed as such. There can be no assurance that an investment strategy will be successful. Historic market trends are not reliable indicators of actual future market behavior or future performance of any particular investment which may differ materially and should not be relied upon as such.
The Hedge Fund Journal, Feb/Mar 2019 Issue 139
Article excerpt republished with permission of THFJ
By Hamlin Lovell
Millburn Machine Learning Continues To Perform
As always, Millburn’s process aims to combine the firm’s substantial market knowledge — decades of experience systematically trading global futures and FX markets — with techniques to understand data. Today, though, the process is distinguished by the application of powerful machine learning technologies (sometimes referred to as statistical learning) — tools more commonly used for single corporate securities — to macro markets. And far from an overlay or sub-strategy, the approach is used holistically for signal generation in the form of both return and trade cost forecasting.
Getting more out of the data
The move in 2013 to the ensemble multi-factor, statistical learning-based framework, blending a variety of models, was therefore less a move away from something than a move towards what the firm saw as a better technology to deploy and harness the growing range of data that had already been feedstock for the models. “Over the last decade, it became clear to us that the explosion in data had the potential to have a major impact on understanding markets. This meant simple rules-based approaches to using momentum were perhaps not going to work as well going forward…we made the decision early-on to remain on the hunt for alpha — which meant investing heavily in a framework that could make better use of a range of data, both price and non-price. But we also wanted a process that had a built-in ability to adapt, to better match the increasing pace of change we were seeing in markets. We saw this as the source of our edge,” says Barry Goodman, Millburn’s co-CEO and Executive Director of Trading.
Re-categorization
“We are looking to deliver a certain type of performance for our investors. In general, we are seeking alpha that is non-correlated to other investments in an investor’s portfolio, so the diversifying characteristic is key. But we are also trying to build a strategy that does not rely upon a particular market environment to have the chance to profit,” he continues.
This has been borne out historically, where the real differentiation in the firm’s return stream has come during non-trending or “choppy” markets. Here Millburn has been able to generate some very good results. And during reversals Millburn was able to minimize losses. This has been crucial because since 2013, markets have been in non-trending environments about 80% of the time, according to Millburn’s classification.
Statistical learning holistically applied
Machine learning is the answer, but it comes in many flavors. In line with the firm’s approach to diversification, the statistical learning techniques used vary across models, and in general no preference is given to a particular technique.
“There are practically unlimited ways to apply machine learning. Different techniques, different training sets, different data inputs, different algorithms within each technique, different retraining frequencies, etc. So there is very much an art of application. This is not ‘cut-and-paste’. We have been working on this for more than seven years and take a very risk-managed, diversified approach — I think that’s been the key to our success to date, versus others who have tried to use these statistical approaches in a deep way,” says Goodman.
Some managers may apply machine learning only to specific sleeves of portfolios or certain functions. In contrast to those who may use machine learning techniques to pick signals or weight standalone models, and in contrast to others who may use the technology only to execute more efficient trades, Millburn applies its multi-factor approach fully to return forecasting and execution cost forecasting; 100% of each forecast comes from these machine learning techniques.
Non-price data inputs growing
“Research into new factors, or ‘features’ in machine learning parlance, is one of the most important dimensions of our process. We’ve been adding new factors at a steady rate since 2013, and today use roughly six times the number we used in July 2013,” says Smith.
In a typical model, price data might constitute 40% or more of the inputs, reflecting the firm’s continued belief in price as potentially good information for return forecasting. Non-price data, however, represents a large and growing proportion of inputs in a typical model. This could include more traditional fundamental or market structure data, for example, but increasingly the firm is evaluating the effectiveness of so-called “alternative” data sources.
However, in certain senses it is slightly artificial to split the data between price and non-price, as each is conditioned by the other – and both can be context- or regime dependent.
Data: quality over quantity
Millburn also thinks that the distinction between traditional and alternative data will become blurred over time. The firm is approached regularly by vendors who have taken what was once “unstructured” data — such as satellite imagery — and turned it into something structured that can be evaluated by their framework. Millburn expects this trend to continue, providing more and more datasets to evaluate. Given the exponential increase in the volume of data available globally, to have used six times as many inputs as five years ago may not be as dramatic an acceleration as it sounds. The expansion of data inputs at Millburn has taken place at what the firm would describe as a “measured pace” because this growing library of datasets has to be carefully curated. For instance, some datasets have been rejected as they may not have long enough histories, or even if long enough the histories may not be comparable and consistent over time. “For us it is all about data, but less about volume than about quality. We don’t worry too much about whether a data source is labeled traditional or alternative. We’re concerned with things like: is the data clean; can it be verified; do we have enough history to let the models ‘learn’ on; but most importantly, is it valuable to the return forecasting process. These are all relatively straightforward criteria for us to test,” says Smith.
While the firm is tight-lipped about the exact nature and volume of data used, they make a point of avoiding the “big data” label. “Ours is not an approach whereby we give the machine hundreds of thousands or millions of esoteric data inputs and just let it run. We’re using data sources that in most cases, were I to show you the list, you would think seem quite reasonable in terms of having a potential influence on returns. Outside of the momentum inputs, which we have been using for decades, many of these data sources have been used at Millburn for ten years or more. So, we’re pretty confident they can add value. The difference in the framework we’ve been using since 2013 is in the contextual approach,” says Goodman.
Contextual quant, noise and human input
The contextual approach, Millburn believes, is a better way to extract alpha out of information. “The whole idea of contextual understanding of markets is incredibly intuitive to us as researchers, and also, it turns out, to our investors. I don’t think anyone would argue that the release of a crude oil inventory number may have some potential impact on near-term price movements in that same market. But perhaps an inventory number released in July may mean something different than if that same number is observed in April, because of heating seasons and driving seasons. And what else might influence the price? Perhaps the price might react a certain way to an elevated inventory number released in April in the context of a surging equity market, as opposed to a falling equity market,” says Smith. Sentiment, seasonality and momentum data could also be combined in various ways. “So, while the interrelationships of data make intuitive sense, the question has been how to tease out these relationships, or influences, in a robust, scientific way. The framework we have built is engineered to do precisely this,” continues Smith.
But this is not easy, especially in the context of financial markets. “Solving for return forecasting in financial markets is quite different to predicting what movie you may like on Netflix, or to predicting what book you may like to buy on Amazon. Financial markets are extremely noisy, and extracting a signal from this noise can be more difficult compared with other industries. It requires real market knowledge, in our view, and experience across many dimensions of investment,” says Goodman.
Parameters of algorithmic techniques need to be more bespoke, and are combined in a unique way to obtain a robust forecast. Though machine learning is now 100% behind the models, this is not a black box approach. It is supervised by human discretion. “Humans need to define inputs and parameters including risk tolerance, and assess data quality, before you flip the switch and let the machine try to find relationships. But it would take too much trial and error for humans to identify complex and conditional multi-factor and multi-data interrelationships that a computer can identify much more quickly and accurately,” says Goodman.
Dynamic resizing and transaction costs
While the risk budgeting and setting of the model parameters are done by the researchers (with the help of quantitative tools), 100% of the active signals and active trading in a market are a direct result of the statistical learning process, in a true hands-off fashion. Within a framework of relatively stable risk budgets per market, the statistical learning processes take over, making near real-time determinations of how much of that risk budget to utilize. The process combines raw return forecasts (generated from an ensemble of learning models in each market) with execution cost forecasts, in order to determine ex ante whether to take a trade, hold off, or perhaps break the trade into pieces. “Signals are only executed when the system thinks there is an opportunity for profit after transaction costs. This can mean deeper, more liquid markets may have a somewhat lower hurdle and trade more frequently, while less liquid markets may more often be governed by transaction costs,” says Smith.
While far from high-frequency, Millburn is sampling data and adjusting signals and return forecasts continuously, 24 hours a day, in light of the stream of new data. Straight-through Processing (STP) allows orders to be transmitted directly to executing brokers, and helps to handle larger volumes of trades as research has found benefit in increased signal frequency. Of course, transaction cost predictions, which also rely on a full machine learning framework, can vary significantly — depending on time of day, depth of the order book, and other conditions considered by the models. While the costs themselves are important, also key is the ability of the systems to predict them accurately.
The result is a strategy that might be best described as “nimble”, focused on a “dimmer switch” approach to staying as close to optimally positioned as possible at any particular time. When investors examine movements in positions, while holding periods still tend to be in the 20-day range on average, it is not uncommon to see positions move from long-to-short or vice versa over the course of even a few days.
Investment universe, diversification and risk control
“These are incredibly powerful technologies, but we are humble in how we apply them. Our goal is not to over-engineer or over-optimize. Risk management is critical for us, and we still believe one of the most reliable ways to control risk is through diversification. So putting the portfolio together starts with what we think is a thoughtful but relatively simple risk budgeting framework, trying to roughly equalize risk allocations across the traded sectors for each program and then, within each sector, across the relevant tradable markets. Staying within the bounds of these budgets helps,” says Smith. Because of the diversification of models and signals and because of the “dimmer switch” approach to trying to stay optimally positioned in every market rather than making binary shifts, generally, the firm will have some nonzero position in each traded market at any time.
The firm’s most diverse programs trade north of 100 global instruments, affording good opportunities for diversification but also, given each instrument can trade multiple times per day, many chances to make successful trades. While the firm has to date avoided some of the more esoteric markets like electricity and others, research is ongoing.
Investor communication
As the firm’s strategies have evolved, so too has its approach to communicating with investors. It can be harder for Millburn to explain exactly what has caused positioning to change given the multiple inputs of dynamically varying weights. Craig Gilbert, who has a mathematics background and joined Millburn from Two Sigma in 2011, just as Millburn’s research turned towards statistical learning, notes that the team has worked hard to make these strategies understandable to its investors. “With quant strategies there will always be a certain part of the process that will be opaque. But much of the process — all the elements that surround the opaque bit — can be explained. Understanding these elements — things like risk controls, operational procedures, data sourcing and cleaning, portfolio construction, signal combination — is, we think, just as important.”
To help, the firm once again leans heavily on data, producing detailed visualizations and animations to demonstrate the mechanics, with the goal of demystifying the approach. Investors can also have sight of signals in each market, and can track how these have evolved over time through the current date. And while explaining the precise genesis of particular signals in markets can be more difficult — the “opaque” piece — the firm does have tools at its disposal to help do this on a case-by-case basis.
But the magic lies partly in the mystery. “By definition, statistical learning approaches seek to uncover patterns or tendencies in historical data more efficiently than could be done by humans. In some cases, these techniques are uncovering real, robust, statistically-significant patterns that would have been entirely impossible for a human being to uncover. So, our natural desire to explain the ‘why’ somewhat misses the point,” says Gilbert. In addition, even with a certain amount of opacity baked-in, most investors take comfort in the process being grounded in some degree of intuition, while understanding that at some point the machine will take over and do the heavy lifting. “Our researchers tend to worry less about why we are positioned a certain way, because of the confidence they have in the process. For investors, because the inputs going into the model are curated and generally quite common sense, we think the trust in the output should also be higher compared with some other quant approaches,” says Goodman.
Orthogonal high-impact hiring
Millburn’s asset growth and strong performance clearly make it attractive as an employer and recruitment has also risen to meet the new bars. The approach to talent at Millburn cuts across all areas of the firm. “We seek smart people, of course, but also place a great deal of emphasis on the right cultural fit. From a research point-of-view, what is common today, and what differs from even ten years ago, is that every hire comes in to the modeling team with strong skills in mathematics and statistical analysis, and the ability to leverage machine learning techniques,” says Gregg Buckbinder, Millburn’s President and Chief Operating Officer. While Millburn’s talent team is often recruiting from some of the same universities as those toured by the likes of Amazon and Google, or even some of the firm’s quant brethren, researchers at Millburn can potentially be much bigger fish in a smaller pool. “We think we offer an environment where a researcher can have somewhat more immediate and direct impact…this is not an environment where a new researcher will find themselves working on projects to eke out marginal basis points,” says Smith. But precisely because each team member can have a major impact, hires are very carefully chosen. As Smith elaborates, “For hires in Research and Development, while we are always looking, we hire very selectively. Our preference is for adding talent with skill-sets that may be orthogonal to those we already have, and/or that can be leveraged in multiple ways by the existing team. Because we operate in a no-silo environment, we think this approach makes sense.”
Millburn also invests heavily in technology. Chief Technology Officer and co-Director of Trading Manu Kambhampati, who joined the firm more than 11 years ago, seeks to keep the firm on the leading edge across all aspects of the business. Says Kambhampati, “in everything we do, we focus on building a highly-resilient, scalable, zero-downtime environment. That’s what our process demands today. Sometimes this means utilizing ’off-the-shelf’ products, but other times we will build customized packages to suit our needs. Like our signaling process, everything is context-specific.” This translates to a mix of proprietary and open-source packages, many of which are highly and specifically modified.
And it has worked. “This philosophy on structuring the R&D team, combined with an efficient research and technology framework, has enabled us to support our growth while avoiding the management challenges that can come with hiring armies of researchers,” says Buckbinder. Assets and capacity Staff have always eaten their own cooking at Millburn. More than USD 400 million, or about 7% of the firm’s AuM, is that of current and former employees, their family members and related entities. These assets are, wherever possible, invested right alongside Millburn’s external investors, without preferential terms with the exception of lower fees, ensuring a good degree of alignment. These co-investments are spread from the most senior staff right down to the most junior, affording each employee the opportunity to invest (company-augmented) retirement pension savings in the firm’s Diversified Program. It’s a perk that is heavily utilized, underscoring the belief that the employees have in their firm. With steady asset growth has come the question of capacity. While the firm demonstrated with the soft closure of its Commodity Program that it is disciplined in protecting its existing investors, in the near term at least it sees no such capacity issues with its main Diversified and Multi-Markets programs. And while Millburn’s CIO Smith is relatively noncommittal, the firm’s current simulations show solid results maintained at levels beyond USD 10bn, even without any allowance for future research to improve scalability. So while not unlimited, there remains significant room to support asset growth – from performance, or net inflows, or both.
The firm’s UCITS strategy, launched on the MLIS (Merrill Lynch Investment Solutions) platform that has now been acquired by Generali Investments, trades virtually pari passu with its Diversified Program, providing exposure to each of the four sectors of equities, currencies, fixed income and commodities. Given the liquid and diversified nature of the strategy, among other things, only minor modifications were needed to be made to accommodate UCITS regulations, meaning tracking error to the flagship is practically nil.
While the firm has always served a wide array of investors, institutions have started to invest more heavily, including some of the largest and most sophisticated pension funds and insurance companies. “These investors have been attracted to our returns, of course, but also to our stability and longevity, and to the firm’s ability to adapt to stay ahead of the competition,” says Goodman. And while Millburn’s US investor base continues to account for the lion’s share of its AuM, interest (and assets) have also been growing from Europe, Asia and Australia as the firm’s brand has become more visible, aided by the tailwind of strong performance.
Outlook: new office, new models and new markets
There’s a certain electricity at Millburn today, as the team moves into bright new offices in Midtown Manhattan, at 55 West 46th Street, just off Avenue of the Americas and four blocks north of Bryant Park. From a research perspective, the framework continues to provide a multitude of opportunities and research is progressing along the three dimensions of additional factors, additional statistical learning techniques, and improved execution.
From a new markets point of view, the firm has entered into joint ventures with local Chinese quant managers to help expand their futures offering for mainland RMB investors. This strategy started with the simple application of Millburn’s international models to the local Chinese futures markets. Performance has been strong and regulatory changes mean this program soon may be accessible by non-Chinese investors. Moreover, some of the local China futures markets themselves are being made available to international investors— for example Iron Ore (traded on the Dalian Commodity Exchange) and Crude Oil futures (traded on the Shanghai International Energy Exchange) were both opened to foreigners in 2018. Millburn expects this trend to continue and its experience trading these markets locally will, they believe, give them a head-start on trading of these instruments in their international programs.
And on the horizon is the application of these models to tactical equity security trading, in the form of a family of tactical sector-based approaches and, ultimately, single-name equities — for the moment just a glimmer in the eye but a promising one. Proof-of-concept has been established and discussions with strategic investors are already underway. Millburn’s unique heritage, culture and process could provide fresh and differentiated perspectives for the quantitative trading of equity and credit. “The firm has come a long way, but we feel like we are only in the early innings,” says Goodman. ●
***
IMPORTANT DISCLOSURES
Millburn’s China Futures Program is implemented through a joint venture entity based in Shanghai, China; Millburn is a shareholder of that entity.
The information provided is accurate as of the date indicated and may be superseded by subsequent market events or for other reasons. Charts and graphs provided herein are for illustrative purposes only. The information in this presentation has been developed internally and/or obtained from sources believed to be reliable; however, neither Millburn nor the author (if not Millburn) guarantees the accuracy, adequacy or completeness of such information. Nothing contained herein constitutes investment, legal, tax or other advice nor is it to be relied on in making an investment or other decision.
This presentation should not be viewed as a current or past recommendation or a solicitation of an offer to buy or sell any securities or to adopt any investment strategy.
The information in this presentation may contain projections or other forward-looking statements regarding future events, targets, forecasts or expectations regarding the strategies described herein, and is only current as of the date indicated. There is no assurance that such events or targets will be achieved, and may be significantly different from that shown here. The information in this presentation, including statements concerning financial market trends, is based on current market conditions, which will fluctuate and may be superseded by subsequent market events or for other reasons. Performance of all cited indices is calculated on a total return basis with dividends reinvested. Neither Millburn (nor any author other than Millburn) assumes any duty to, nor undertakes to update forward looking statements.
The performance and other information is based on that which is available as of the date of this report. Any markets, models, leverage, portfolio weights and other data or statistics described change over time, but are accurate as of the date indicated herein.
RISKS OF AN INVESTMENT IN EACH MILLBURN PROGRAM include but are not limited to the following: (i)The Program is speculative. Investors may lose all or a substantial portion of their investments in the Program. An investor in an Account that is not structured as a limited liability vehicle may lose more than the amount of its investment; (ii) The Program is leveraged. The Program will acquire positions with a face amount of as much as eight to ten times or more of its total equity. Leverage magnifies the impact of both profit and loss; (iii) The performance of the Program is expected to be volatile; (iv) Investors will sustain losses if the Program is unable to generate sufficient trading profits and interest income to offset its fees and expenses; (v) A lack of liquidity in the markets in which the Program trades could make it impossible for the Program to realize profits or limit losses; (vi) A substantial portion of the trades executed for the Program take place on non-US exchanges. No US regulatory authority or exchange has the power to compel the enforcement of the rules of a foreign board of trade or any applicable foreign laws.
This information is not an offer to sell any product or a solicitation of an offer to invest or open an account (an “Account”) and must be supplemented by a disclosure document when considering an investment. An Account may be opened only after receipt and review of a disclosure document and execution of certain agreements. An Account disclosure document contains important information concerning risk factors, conflicts of interest and other material aspects of an investment; this must be read carefully before any decision whether to invest is made.
Commodity interest accounts are illiquid, speculative, employ significant leverage, and involve a high degree of risk. Commodity interest products involve high fees. Please see the disclosure document for a detailed description of these and other "Risk Factors" and "Conflicts of Interest." There can be no assurance that an Account will achieve its objectives.